Count lines of code at your own peril: it is the most arbitrary, low-signal metric still widely used
It's conventional wisdom that assessing developers via lines of code (also referred to as "LoC", "cloc" or "sloc") has a long, fraught, history. Managers, particularly those who have written code themselves, loathe the prospect of developer measurement via LoC.
By itself, LoC is an even less precise metric than "commits made" -- itself an awful metric upon which to base any important decision. Like almonds and cashews, LoC begins its life as a poison unfit for human consumption.
If extracted with careful precision, that signal can be used to clarify how code is evolving, which can in turn answer essential questions that are otherwise impenetrable.
The fight we are up against? That almost all lines of code are "garbage data" from the standpoint of trying to draw accurate conclusions. The research we've conducted suggests that, when you say that LoC is a "useless metric," you're right... but only about 95% right. Capture that last 5%, and you'll have a transformative means to understand the essence of how your repo changes over time.
See also: Data-backed visualization of sloc 95% noise sources
The link above uses empirical data to statistically prove, in an illustrated funnel, how lines of code ends up being 95% noise. To cultivate an intuitive feel for what makes LoC such a dirty signal to draw from, we'll provide a real life analog to lines of code in the article below.
Like counting plates in a restaurant
Conventional wisdom tell us that it's possible to improve business results by improving business measurement. In other words, the thing that we choose to measure is the thing that improves. There's a big opportunity here, but it's bundled with an equally big risk of using noisy LoC data to make the wrong decisions. To illustrate what makes counting LoC so risky, consider this analogy:
measuring developer impact by lines of code
≡
measuring restaurant productivity by numbers of plates used
For our purposes, a A "productive restaurant" is defined as one that "serves as many delicious meals as possible to customers." Using this real world touch point will allow us to explain, in non-technical terms, the major reasons that counting LoC is a proposition teeming with danger.
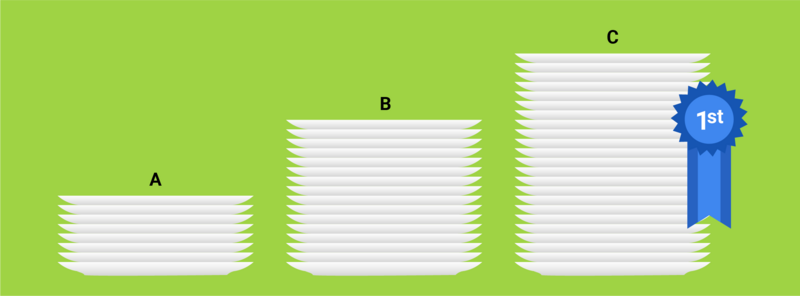
Behold Restaurant C: an indisputable Best Restaurant™ juggernaut
Let's start with some good news for restaurant owners. If they're using a lot of plates, they're probably serving a lot of meals! And if they're serving a lot of meals, they've probably built a strong reputation on Yelp, and could be considered "productive" by our definition. To the extent we can sort out only those plates being used to serve delicious meals, we can analyze how productive your restaurant was over time. Having a consistent metric like "adjusted meaningful plates used" (glides off your tongue) lets us map decisions (e.g., special events, Groupon offers) into immediate feedback. Then you repeat the stuff that worked.
Better still, the The data we collect can allow us to A/B test new techniques & methodologies so that we gradually adopt practices that maximize what we measure. In the world of code, measurement can allow management to visualize how developer impact is shaped by events like meetings, working from home, or pair programming. Good measurement allows good management to experiment with confidence.
There is signal embedded in these roundabout metrics, plates and LoC (collectively, "units"). But you have to want it bad. Real bad. But you have to want it reaal bad.
Surface Problems
The first and most obvious class of problems encountered when tallying units are "surface problems." They represent a failure of our incrementing unit to bring us closer to the result we hoped to measure. In restaurants, there are several practices that expend plates without delivering delicious meals (our gold standard for a productive restaurant).
Example 1: This plate intentionally left blank
Fancy restaurants: so many plates, so little food
At upscale restaurants, it's conventional for tables to be set with empty plates waiting to greet visitors. These plates contribute to the restaurant's plate count, but typically they won't be used for a meal. As such, all of the unused plates set out by convention do nothing to help us understand the restaurant's productivity.
In the US, it's conventional for fancy restaurants to set their tables with empty plates. The plates used on behalf of this convention let us infer nothing of the restaurant's productivity.
Similarly, many code lines get changed by way of language or project convention, adding a lot of noise. These are lines like: whitespace changes (changing word to   word), blank lines, and language based keywords (begin, {}).
- Whitespace changes (changing word to   word)
- Blank lines (to improve readability)
- Language-based keywords (begin, end, {} )
Line changes beget by convention account for a staggering 54 percent of all LoC in commits analyzed on GitClear in 2017 . Devaluing lines that match this pattern gets us a big step closer to identifying the work that made a meaningful impact.
Example 2: Moving from plate to plate
When my wife and I dine out, we enjoy sharing different selections from the menu. We request two empty plates for splitting the meals, as well as several more filled with the various foods we order. We divide these up throughout the meal, moving food from plate to plate. The plates we use to move food around tell the restaurant nothing about how many delicious meals it is serving. But they do increase the plate count.
When my wife and I dine out, we request two empty plates so that we can share our meals. The plates that we use to move food around tell the restaurant nothing about how many delicious meals it is serving, but they do increase the plate count.
In code, it's common for developers to cut and paste lines from one file to another, or from one function to another within the same file. This type of line change happens rapidly, and tells us virtually nothing about the volume of meaningful work happening.
In code, it's common to move blocks of code between files or methods. Our data suggests this comprises about <b>30 percent</b> of all changed lines of code. If your code quantification tool doesn't recognize moved lines, your stats could have 15% noise built into them.
If you use a code quantification service that doesn't recognize moved lines, the signal your measurements contain is being diluted by about 30 percent, the average percentage of "moved lines" among all LoC within GitClear repos.
Example 3: The plates you never see
The pristine result we're presented when our server brings out dinner is often a far cry from the laborious mess that went into preparing the meal. In the course of cooking, chopping, and mixing, an untold number of plates may be consumed by the restaurant on behalf of creating the idyllic end product that you chow down on. The count of plates that reflect restaurant productivity should exclude all such plates to maximize accuracy.
The dinner plate we receive at a restaurant may only be a fraction of the plates used to prepare the dinner. We maximize accuracy by negating the value of behind-the-scenes plates.
However many plates are wasted behind the scenes at restaurants surely pales in comparison to the scores of lines discarded by developers in pursuit of a concise feature implementation. By the time the feature is ready to be shipped, most of initial LoC for a two week feature has been rewritten. In contrast to our friends at Pluralsight Flow , we don't consider this churned code a meaningful signal to worry about unto itself; our experience has been that the percentage of code that a developer churns is independent of the quality of their work, or the speed at which it's completed. But churn should be recognized and deducted during the calculation of developer output.
Experienced developers will often sketch out a quick & dirty first-pass implementation without regard for clarity or quality. By the time the feature is ready to be shipped, all that initial LoC has often been revised and rewritten. Churn should be recognized and deducted during the calculation of developer output, since it doesn't reflect valuable work done until it reaches its final state.
Among all LoC we processed in 2018, about 11 percent of lines were "churned," meaning that they were replaced or removed a short time after having been initially committed.
Structural problems
Even when both plates hold a meal, that doesn't make them equally delicious
The second class of concerns can be considered "structural" in nature. They include endemic factors (e.g., good location) that impact the rate of plate usage independently of the restaurant's productive output. In contrast to "surface concerns," structural concerns don't change the tally of units (plates or LoC) included in our calculation. Rather, they imply a scalar should be applied to the unit count to reduce the impact of irrelevant externalities.
Example 1: Location is everything
Many restaurants live or die based on their location. If your restaurant is next to the hottest tourist destination in town, it's nigh inevitable that you'll end up with a lot of meals ordered. Does that mean that you're a productive restaurant? Not necessarily. In order to compare your result in the context of similar restaurants in different locations, we must dilute the credit we ascribe to your plates used. Your location dictates that your plate count will be relatively high regardless of whether you're serving delicious meals. In this case, a high number of meals ordered doesn't directly correspond to the restaurant consistently serving delicious meals. Its productivity is to be scaled down on behalf of the natural tendency to succeed.
In code, certain languages naturally produce more LoC than others. Verbose languages like HTML and CSS, a developer's output will appear prolific, especially when implementing a new feature. In contrast, concise programming languages like Python, Ruby, or C# will naturally end up with fewer LoC. PHP, Java and C++ fall somewhere in between. GitClear analyzes all of these languages, so it's essential for us to help make them "apples to apples" when viewed together in the same graph.
GitClear makes it easy to customize Diff Delta for different languages and file types. Managers can adjust the multipliers that our learning algorithm applies to match the languages & conventions used by the project. For example, we've found that a line of CSS has about 40 percent of the impact as a line written in a concise language (e.g., Python, Ruby). Put another way, we expect that a developer writing CSS will produce about 40% more LoC than that same developer writing Ruby. God only knows how much they could get done in one line of Lisp.
Example 2: Cheap food begets more meals ordered
Say you run a restaurant with the cheapest burgers in town. You serve a high number of meals each day, so our count of your plates used to serve meals is high. But this only tells us half the story. The other half of our "productivity" definition is that you're serving delicious meals. The high rate at which you're using plates tells us nothing about the quality of the food you're serving on them. If anything, the fact that your burgers are cheap probably correlates negatively with deliciousness. Our evaluation of your restaurant's productivity will best reflect reality if we reduce the value per plate based upon your great location. But we have no idea if the meals you serve are "delicious" (half the definition of "productivity"), so your plate count needs to be reduced such that it doesn't give undue credit for price.
Most every developer has worked on a team with someone that creates volumes of “cheap” lines of code. When a developer copy and pastes code, they add lines that serve a purpose and get the job done. But rarely is that code considered to have a positive impact. It repeats existing code and becomes a hassle when it needs to be changed in the future. When code is copy/pasted or find/replaced, we need to reduce our interpretation of the impact conferred.
If we're measuring developer contributions, we shouldn't reward this type of "cheap" code – even if it leads to successfully implementing a feature. That's why we identify copied and pasted, as well as find / replaced code to reduce their Diff Delta.
Example 3: Bowls on the loose
Just when we thought we'd accounted for all the nuances of plate usage, and now this: it turns out that some meals get served in bowls ;-(. And dessert plates. And trays. This is terrible, terrible news. If we want our plate count to fully and accurately depict the productivity of our restaurant, we need to know how much of our volume takes place in each type of vessel.
In code, the "classic" line of code implements a feature (or fixes a bug). But what about LoC that serve as the documentation for a feature? What about the tests that are implemented in support of the feature? A rounded metric should define an equivalency between LoC that created for various purposes, so that if we find developers are able to write tests or documentation more quickly than "classic" code, we adjust the value of these LoC accordingly.
And lest you think "documentation" and "tests" aren't a major contributor to your total LoC, they are but the tip of the iceberg. Consider: auto-generated code, language inserted in view files, and code added as part of third-party libraries. Add up all the non-classic forms of LoC, and you have about 70 percent of the lines in a typical repo. We allow you to define & detect as many custom types of code as are applicable to your project.
Consider how many different "categories" of code exist within your repo. There's "classic" code that implement a feature. Then there is: documentation, test harnesses, third-party libraries, and much more than we can cover in a short story. About 70 percent of LoC in a typical repo is comprised of code that isn't "classic." It should be worth something, but that perfect scalar varies by code category.
Better measurement via Diff Delta
If you've made it this far, you've learned the major reasons it's so difficult to use LoC to measure a developer's impact. Almost all LoC is "noise" from the standpoint of whether it reflects a lasting impact made to the code repo; this explains why any naive interpretation of LoC will serve to reliably aggravate sophisticated managers. They recognize how little traditional LoC tools (or GitHub's "Insights" tab) correspond to meaningful work completed.
It's only through tenacity and attention to detail that one can methodically catalog all the ways that LoC misleads. It took our team about 30 months of concerted pre-launch effort to build a system that that can sift through all the Surface and Structural problems with measuring LoC and consolidate them into a single metric.
We call this metric Diff Delta, and we believe that it's the most accurate option for teams who want to measure the rate at which their development team is evolving the repo.
Much of our development time has gone toward ensuring that individual teams can adjust the scalars we apply to LoC in different code categories, file types, and so on. We've worked hard to ensure that our default values are sensible for general use cases – this isn't a tool you'll need to spend hours configuring before you begin to take away usable insights. But we also appreciate that reasonable CTOs can come to very different conclusions of how much impact is made by writing a test, writing a line of CSS, or updating a file annotation.